The Secret to Securing Enterprise Data While Using Generative AI? Just Ask Guardian.
January 28, 2025
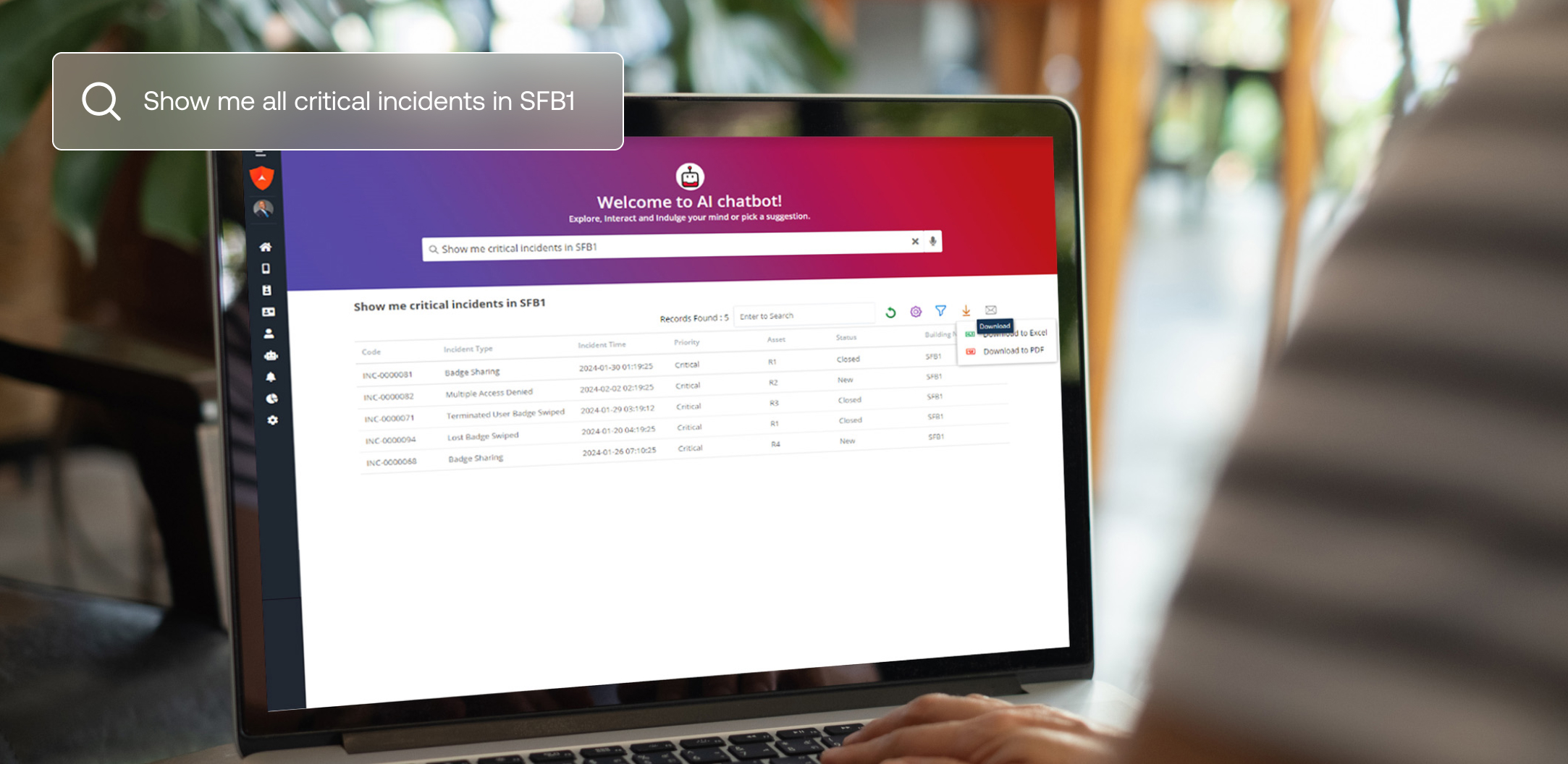
Harness the power of generative AI to improve efficiency and reduce costs without compromising data security
Generative AI is becoming mainstream, embedding itself into enterprise business processes and promising optimized operations and cost savings. However, concerns about exposing sensitive data to Large Language Models (LLMs) have made organizations hesitant to adopt the technology – especially when it involves critical information about their processes, technology and users.
A recent Cisco survey found that more than 1 in 4 organizations has banned generative AI due to privacy and data security concerns. This highlights the significant tension between the desire to innovate with AI and the critical need to protect enterprise data.
Exploring Data Exposure Risks
Organizations face unique challenges in safeguarding two types of data:
- Structured Data: Stored in relational databases as tables and columns
- Unstructured Data: Found in documents, policies, processes, images and knowledge articles
LLMs require access to data to learn and generate contextually relevant outputs. However, exposing enterprise data to LLMs introduces additional risks, as illustrated in these scenarios:
Scenario 1: Sensitive Business Data Exposure
Imagine an enterprise ERP vendor using LLMs to assist with NLP-based invoice generation. By providing sensitive data like part numbers, unit pricing and discount policies, the organization risks indirect exposure. If another user asks, “What is the typical discount for nonprofits?” it could inadvertently reveal sensitive insights learned by the LLM.
This data exposure problem occurs when LLMs unintentionally use learned information outside its intended context, leading to potential business risks.
Scenario 2: Compliance Violations Across Geographies
Organizations operating across multiple regions must adhere to stringent data privacy and localization regulations. For instance, European employee PII cannot be shared outside Europe. Exposing such data to LLMs could violate data processing agreements and breach regulatory and compliance boundaries.
Emerging Threats and Vulnerabilities
As generative AI evolves, so do its risks. Early adopters must consider vulnerabilities across infrastructure, networks and applications. Some notable risks include:
- LLM Data Leaks: Unintentional revelations of sensitive proprietary information
- Prompt Injection Attacks: Manipulative queries designed to extract confidential data
- Model Theft: The unauthorized access or tampering of LLM models
Current Tools and Their Limitations
While LLM providers and third-party companies offer tools for data security, these solutions primarily focus on unstructured data (like documents). They often fail to address the root issue of structured data exposure.
For example, data-masking techniques can obscure sensitive information during LLM training. However, they do not prevent LLMs from inadvertently learning trends or averages with the potential for misuse across organizational boundaries.
A Holistic Solution: Alert Enterprise’s Approach
The most effective way to secure enterprise data is to entirely prevent LLMs from accessing actual structured data. Alert Enterprise has developed a patented technology to achieve this by exposing LLMs only to metadata, such as table structures and entities. The LLM uses Natural Language Processing (NLP) to convert queries into metadata, which the application then translates into machine-readable syntax to fetch data securely.
Our Guardian Security AI Chatbot is built on this technology and enables users to ask data analytics questions in natural language. The chatbot provides instant, accurate responses without compromising sensitive data.
This innovative approach ensures:
- LLMs never access raw data, which eliminates exposure risks.
- Enterprises retain full control over their structured data, maintaining compliance across regions and departments.
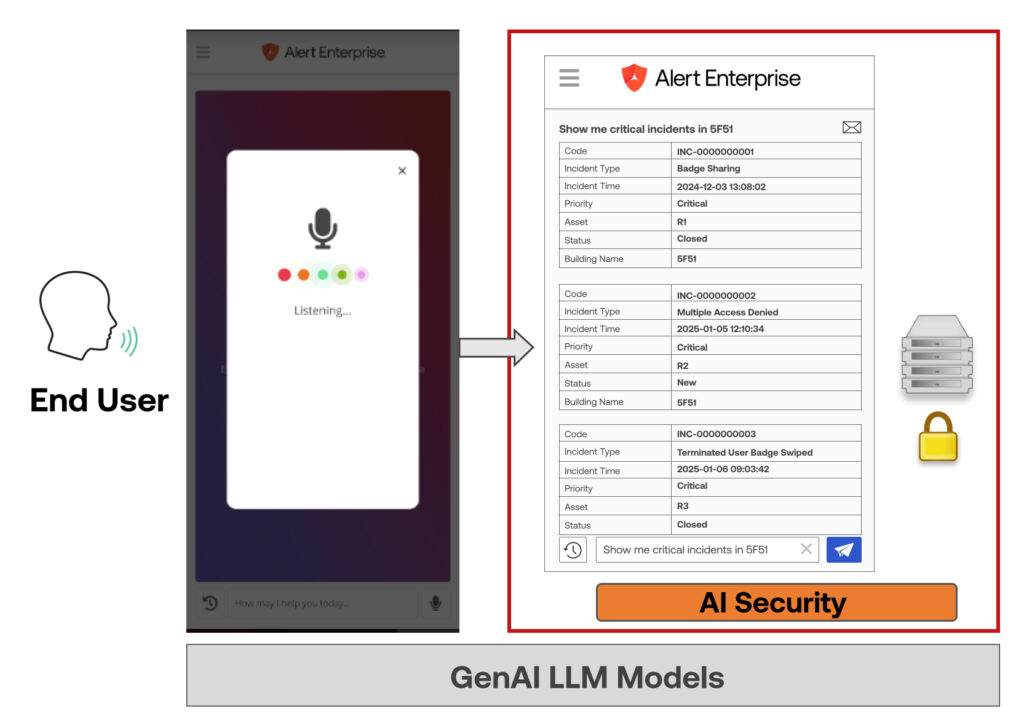
Want to learn more?
Explore our groundbreaking generative AI data privacy patent here.